Artificial Intelligence (AI) Career Roadmap: Jobs and Levels Guide
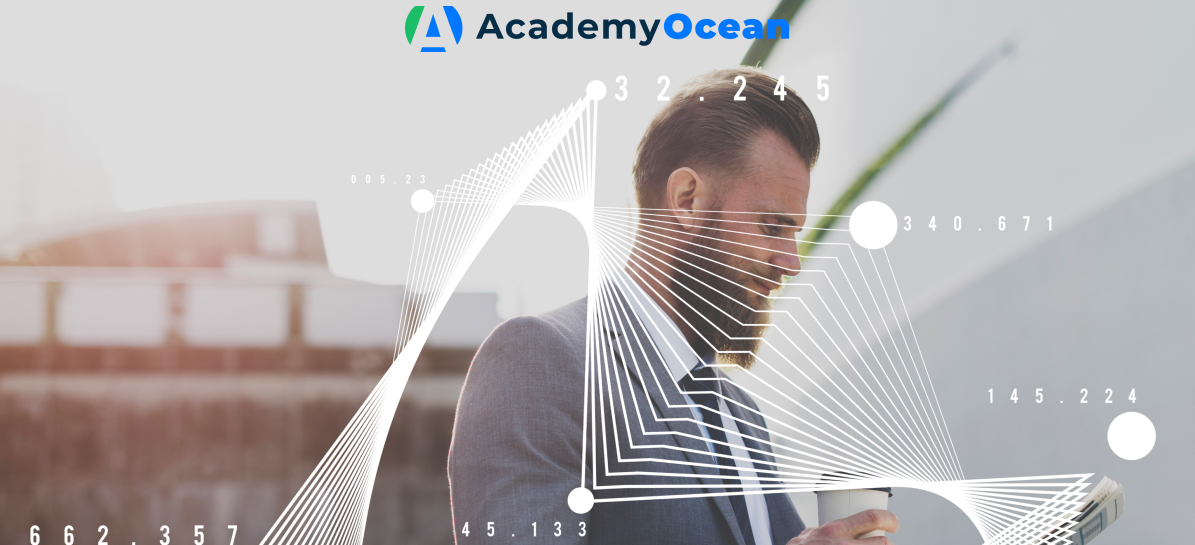
Decoding the Hierarchy: The Different Levels of AI Careers
Mapping the Terrain: Common AI Roles and Career Paths
Your Launchpad: How to Start a Career in Artificial Intelligence
Charting Your Course: Roadmap to Learn AI and Machine Learning
Specialized Routes: AI Career Pathways in Machine Learning and Deep Learning
Avoiding Potholes: Common Mistakes in AI Career Development and How to Avoid Them

The world of artificial intelligence hums with possibility, especially in 2025. While AI is promising innovation and new opportunities for younger generations, it threatens longly established professionals.
Wherever you stand, understanding the AI Career Roadmap will be very beneficial for your aspirations. Surely, you can use an advanced AI career path generator and build your career. However, a deeper understanding of the mater is more than just about saving your job or getting a new one. It is about being a part of the processes shaping the world.
Decoding the Hierarchy: The Different Levels of AI Careers
Luckily, AI professionals, like those in any mature industry, follow a predictable path, from basic responsibilities to strategic leadership. However, every level requires its own emphasis, duties, and skill set.
Entry Level
Initially, these jobs entail data annotation, model testing, or assistance with fundamental workflows. All this serves to help beginners understand and adapt to the AI lifecycle and get experience.
Mid-Level
With expertise, individuals advance to more specialized roles such as data scientists, machine learning engineers, and AI developers. These roles are more sophisticated and entail constructing, improving, and deploying models. Therefore, advanced technical knowledge becomes a clear must at this level.
Senior & Leadership Roles
Strategic jobs at the top include AI leaders, directors, and AI heads. These people are responsible for global planning and control. Thus, they handle significant projects and coordinate AI initiatives inside corporations.
Mapping the Terrain: Common AI Roles and Career Paths
Machine Learning Engineers build and deploy predictive models.
Data Scientists analyze large datasets to uncover insights.
AI Researchers explore new methods and push the boundaries of innovation.
Just like with typical jobs, your route is determined by your own abilities and interests. For example, a strong math background can bring you to research, in turn good coding abilities may make engineering an obvious choice. However, you must understand that these rules aren't set in stone. It means that real-life situations can significantly differ from the ideal cases.
Additionally, career transitions aren't something new and unusual. For example, a Data Scientist may transition to engineering after becoming interested in model deployment. As your abilities advance and market demands shift, so can your path. Remember, intellectual curiosity and mobility are your best friends.

Launching your career in AI is pretty similar to traditional jobs — it requires planning. You analyze market trends and demands. Then you compare them with your current abilities and find your strong sides and those that require some improvement.
For example, a thorough understanding of arithmetic, statistics, and computer science is a great advantage. However, even without it, you still have chances as you can provide a unique view on problems and tasks.
To boost your chances:
Build a portfolio.
Showcase skills
Take courses or bootcamps
Network and seek mentorship
Charting Your Course: Roadmap to Learn AI and Machine Learning
Take a look at a simplified roadmap
- Learn the basics of programming
- Study core machine learning concepts
- Build your data skills.
- Advance to deep learning & NLP
- Use the right resources
A typical path looks like this:
Intro to Python → ML course (with projects) → Specialization (e.g., NLP or computer vision)
Progress step by step — and build as you go.
Specialized Routes: AI Career Pathways in Machine Learning and Deep Learning
Within AI, two major specializations stand out: machine learning and deep learning.
Machine learning includes developing algorithms that learn from data. The greatest examples are algorithms behind fraud detection systems and recommendation engines.
Deep learning, while being in fact a part of the machine learning, the deep learning is about multilayered neural networks that work n more complicated patters. These algorithms drive image recognition and natural language processing.
Your route strongly depends on your talents and interests:
📌 Interested in business predictions? Choose machine learning.
📌 Passionate about image or speech recognition? Explore deep learning.
Credentials and Criteria: AI Certifications and Job Requirements
Certifications are not always needed, but they enhance your reputation and can help you stand out. Top employers look for:
Solid grasp of ML/AI algorithms
Strong programming skills
Hands-on experience with data
For senior roles, expect:
✅ Proven project success
✅ Leadership experience
✅ Preferred certifications (e.g., cloud platforms, deep learning)
Tip: Always examine job advertisements to align your abilities and certifications with the real-world needs.
Avoiding Potholes: Common Mistakes in AI Career Development and How to Avoid Them
Sadly or luckily, the route to a successful AI profession is not always straightforward. Many professionals fall into the trap of relying solely on academic knowledge and forgetting the importance of hands-on experience. The best option for you to raise your chances of getting such a job is to build a solid portfolio of real-world projects. Regardless of your experience, education, and skills, a portfolio works best for demonstrating your talents and standing out to employers.
Another common mistake is ignoring networking. Remember, you are not alone. Making relationships at industry events, online networks, and professional organizations will definitely help you find career prospects and useful advice.
✅ To avoid these mistakes:
Work on personal or open-source projects
Look for internships or freelance opportunities
Engage with the AI community
Commit to lifelong learning
